Introduction:
Financial engineering has always relied on data-driven decision-making. With the advent of machine learning (ML), the financial industry is undergoing a paradigm shift. From predicting stock prices to risk management, machine learning empowers financial engineers to tackle complex problems with greater precision. In this article, we will explore how machine learning is revolutionizing financial engineering applications and its future potential.
What is Financial Engineering?
Financial engineering involves the use of mathematical tools, statistical methods, and computational techniques to solve financial problems. It plays a critical role in portfolio management, derivatives pricing, risk assessment, and financial forecasting.
With the growing complexity of financial markets, machine learning has emerged as a game-changer, allowing financial engineers to process vast amounts of data efficiently and extract valuable insights.
How Machine Learning is Revolutionizing Financial Engineering
1. Algorithmic Trading:
Machine learning models, such as reinforcement learning, are widely used in developing trading algorithms. These algorithms analyze market data in real-time, identify trading opportunities, and execute trades faster than humans ever could.
2. Risk Management:
Risk assessment is a vital part of financial engineering. ML algorithms like neural networks and decision trees can predict credit defaults, fraud detection, and market risks with high accuracy.
3. Portfolio Optimization:
Financial engineers use ML models to optimize portfolios by balancing risk and return. Models like support vector machines (SVM) and gradient boosting help in analyzing historical data to make better investment decisions.
4. Fraud Detection:
ML-based fraud detection systems monitor transactions in real time, flagging anomalies that deviate from normal behavior. Techniques like clustering and anomaly detection have proven highly effective in minimizing fraud.
5. Pricing Financial Instruments:
Complex derivatives pricing often involves significant computational effort. ML models like Monte Carlo simulations, combined with deep learning, provide faster and more accurate pricing mechanisms.
Popular Machine Learning Models Used in Financial Engineering
1. Linear Regression and Logistic Regression:
Used for predicting stock prices and credit scoring.
2. Random Forest and Decision Trees:
Widely used in fraud detection and portfolio optimization.
3. Neural Networks:
Ideal for analyzing high-dimensional datasets like stock market data.
4. Reinforcement Learning:
Best suited for developing automated trading strategies.
5. Clustering Algorithms:
Used for customer segmentation and credit risk analysis.
Challenges in Applying Machine Learning to Financial Engineering
Despite its potential, machine learning faces several challenges in financial engineering.
Data Quality: Financial data is often noisy, incomplete, or inconsistent.
Model Interpretability: Black-box models like deep learning can lack transparency, making it hard for regulators to approve their use.
Regulatory Compliance: Strict financial regulations may limit the adoption of advanced ML techniques.
Future of Machine Learning in Financial Engineering
The future of financial engineering lies in advanced machine learning models combined with big data analytics. Innovations like quantum computing and real-time data analysis are expected to further enhance ML’s impact on financial applications. Financial institutions will increasingly adopt AI-driven technologies to remain competitive.
Conclusion:
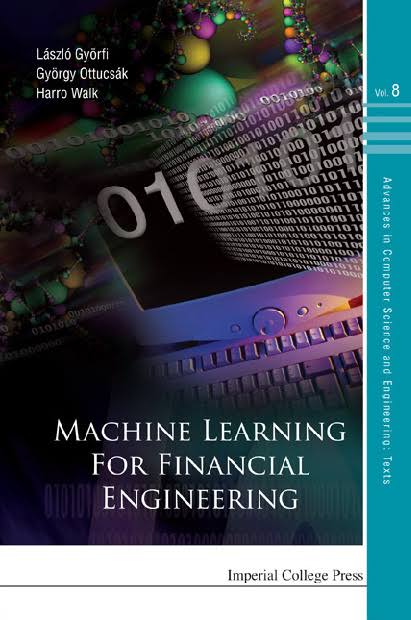
Machine learning is a transformative force in financial engineering, offering innovative solutions to age-old financial problems. From risk management to fraud detection and beyond, the applications of ML are boundless. As the field evolves, financial engineers must embrace machine learning to stay ahead in this dynamic landscape.
By integrating advanced ML techniques, financial engineering is poised to achieve unprecedented levels of accuracy and efficiency.
One thought on “Machine Learning for Financial Engineering Applications”
Comments are closed.